What it is about
In this article, we outline the areas in which AI can help to speed up processes on the shopfloor, increase reliability and quality and improve energy management in production. What requirements do companies need to fulfill in order to implement AI applications and what factors are hindering the use of artificial intelligence?
More than automation: AI algorithms create new knowledge
Automation is not new, but it has been a major step forward for the industry: automation technologies relieve employees of monotonous, repetitive tasks. Automation software therefore helps to increase efficiency enormously and reduce costs. In addition, by automating processes, companies can eliminate human error and protect themselves from its consequences.
Artificial intelligence (AI), on the other hand, is based on self-learning algorithms. They are trained with large amounts of data and thus improve their performance over time. Unlike automation software, they do not perform simple tasks, but complex ones. They can predict developments and make decisions.
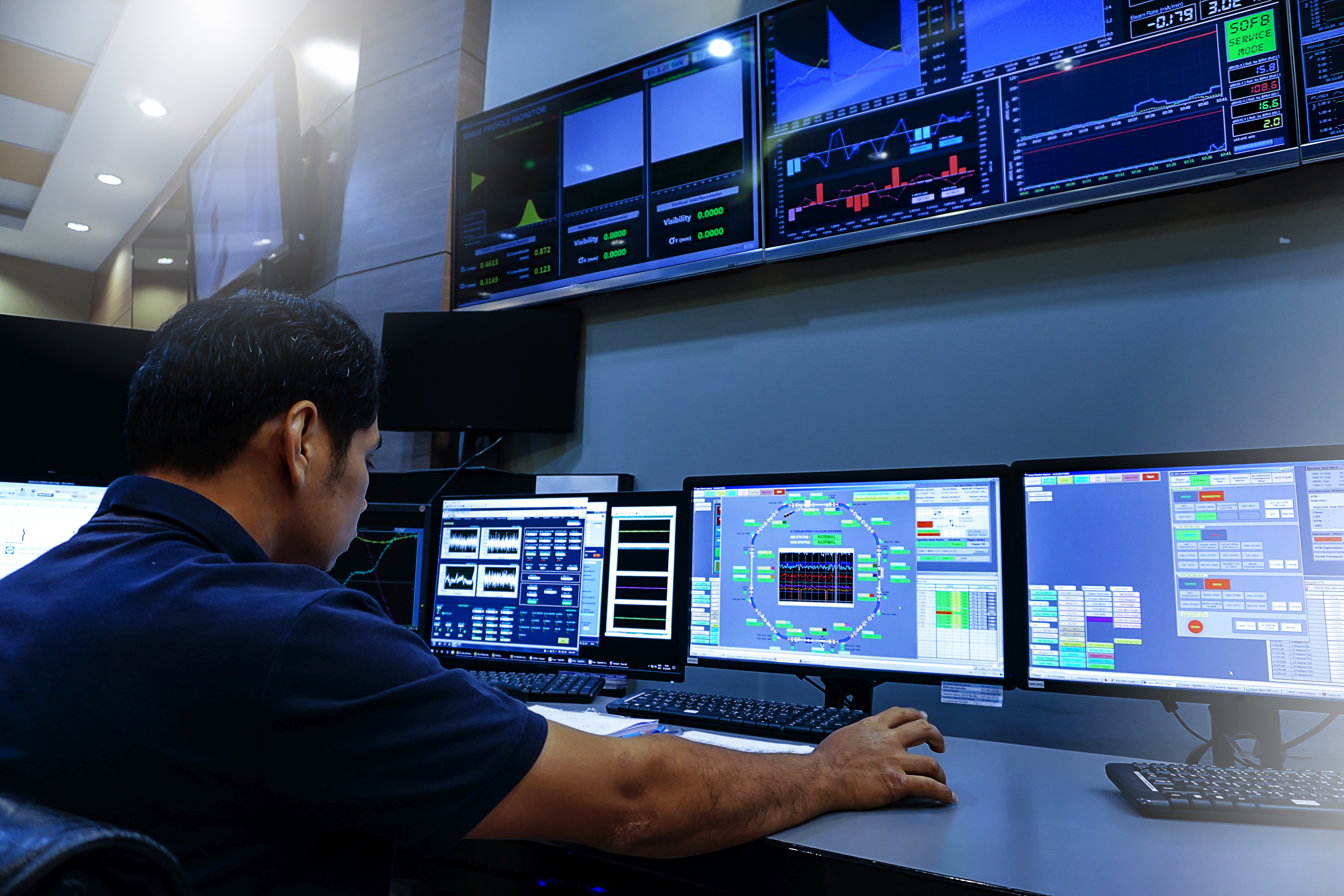
Where AI is already being used in production today
In principle, artificial intelligence algorithms work like the human brain. However, they have a much higher "computing power" and can therefore solve various tasks in production faster and better than humans.
Field of action 1: Commissioning of machines and order processing
AI-based software helps customers in the mechanical and plant engineering sector to commission production machines and process orders. Various processes that guide employees through commissioning can be derived step by step from a digital representation of the production environment, the digital twin. In this way, AI also guides the workers through the entire order processing process, including set-up instructions and inspections. Because the software learns from all events on the shopfloor - assuming they are recorded digitally - companies optimize their production processes over time and can eliminate the causes of errors. Manufacturing companies not only become faster, but also improve their quality.
Field of action 2: Increase productivity with predictive maintenance
The market already offers very sophisticated solutions for predictive maintenance. By analyzing machine data and deriving forecasts from it, AI enables employees in production to recognize at an early stage when the machine needs maintenance, for example when wearing parts need to be replaced. Regular maintenance intervals can also be adjusted based on algorithms. Companies reduce their costs if they increase the intervals without risking downtime. Anomalies are detected long before damage occurs and unplanned downtimes can be largely prevented. This enables companies to increase their productivity and machine availability.
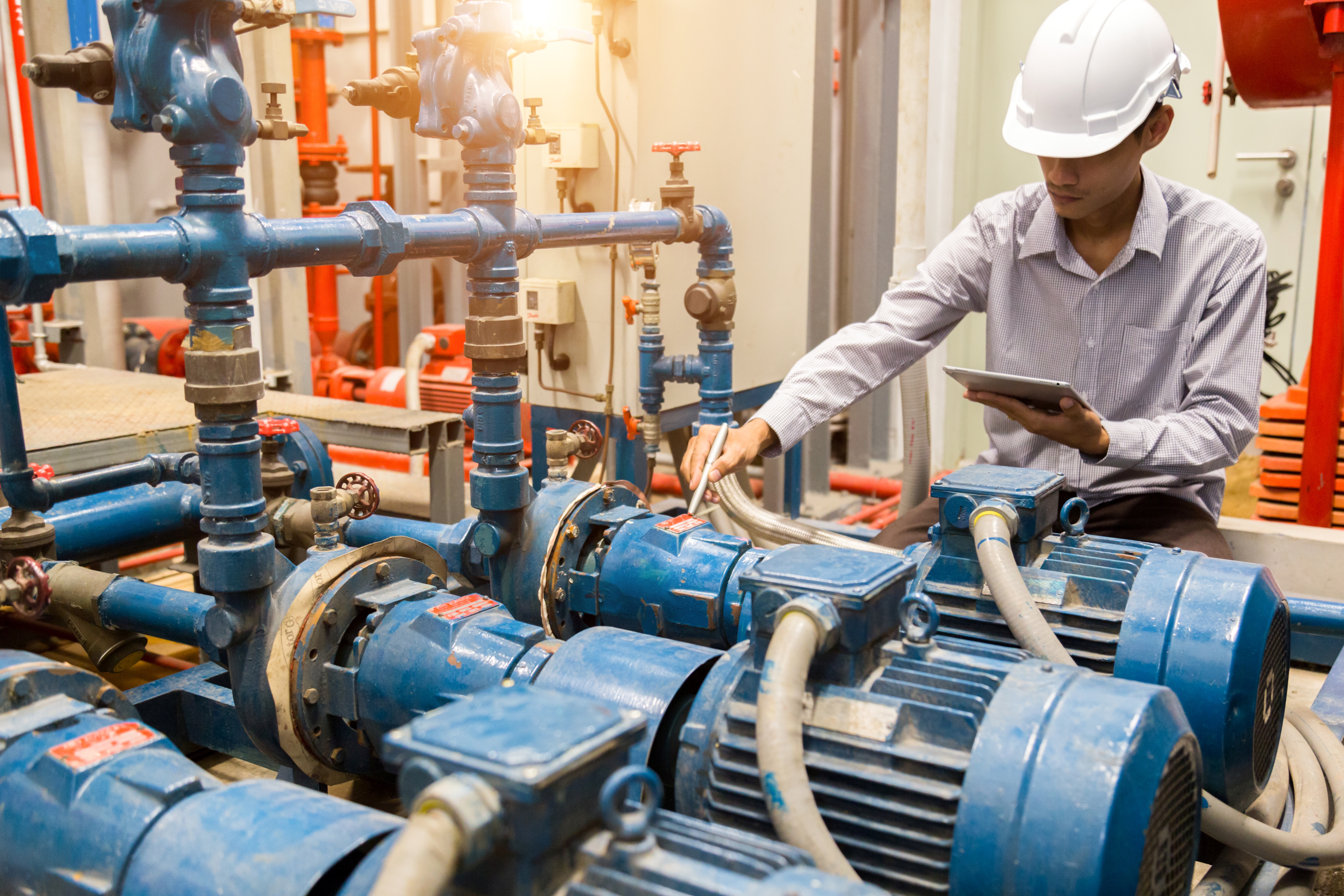
Field of action 3: Check and ensure quality better and with less effort
Quality inspection is indispensable in production. However, it is time-consuming and does not always deliver the desired results with conventional methods. AI expands the scope of action in quality assurance. Without having to damage the product, it can be checked for defects and production errors using visual inspection. Employees can use visual inspections to detect scratches, cracks, casting errors, incorrect positioning of parts and other defects.
It is now possible to carry out camera-supported visual inspections automatically. The software detects the slightest deviations from the good and bad images with which the system has been trained in real time and analyzes the causes. For example, it could be that employees regularly do not carry out a certain work step gently enough. By reporting the error to the employee, the software prompts them to rectify it. The employee learns how to carry out the work step correctly. At the same time, costs are reduced because the errors are not only detected during quality control of the finished part. As the overall product quality increases through the evaluation of the AI, customers have less cause for complaint - an important contribution to customer loyalty and cost reduction. The automated process increases productivity by reducing waste. In addition, skilled workers gain capacity for other demanding tasks.
Field of action 4: Energy management
Companies are required to use energy sustainably. In doing so, they not only reduce their costs, but also align their processes with ESG goals, where ESG stands for environment, social and governance. With the sustainability reporting obligation and other requirements, legislators are setting standards that businesses must meet. It is therefore advisable to integrate energy management into the production environment. As there is often a lack of awareness of energy efficiency on the shopfloor, comprehensive, self-optimizing systems help to achieve a better energy balance. They often enable energy guzzlers and inefficient behavior to be identified and eliminated for the first time.
What makes the introduction of artificial intelligence difficult
A look at manufacturing practice shows that AI-based processes are still a dream of the future for many companies. The biggest hurdle is making data available and consolidating it. For example, energy data is usually recorded in separate systems and does not easily find its way into business management software or an MES system. An algorithm is only as good as the data it is fed with. In practice, data quality proves to be the key shortcoming for AI projects. Unfortunately, it is not enough for energy, performance, quality and so on data to be collected at all; it must also meet the quality standards of the software and be able to be processed in a uniform manner.
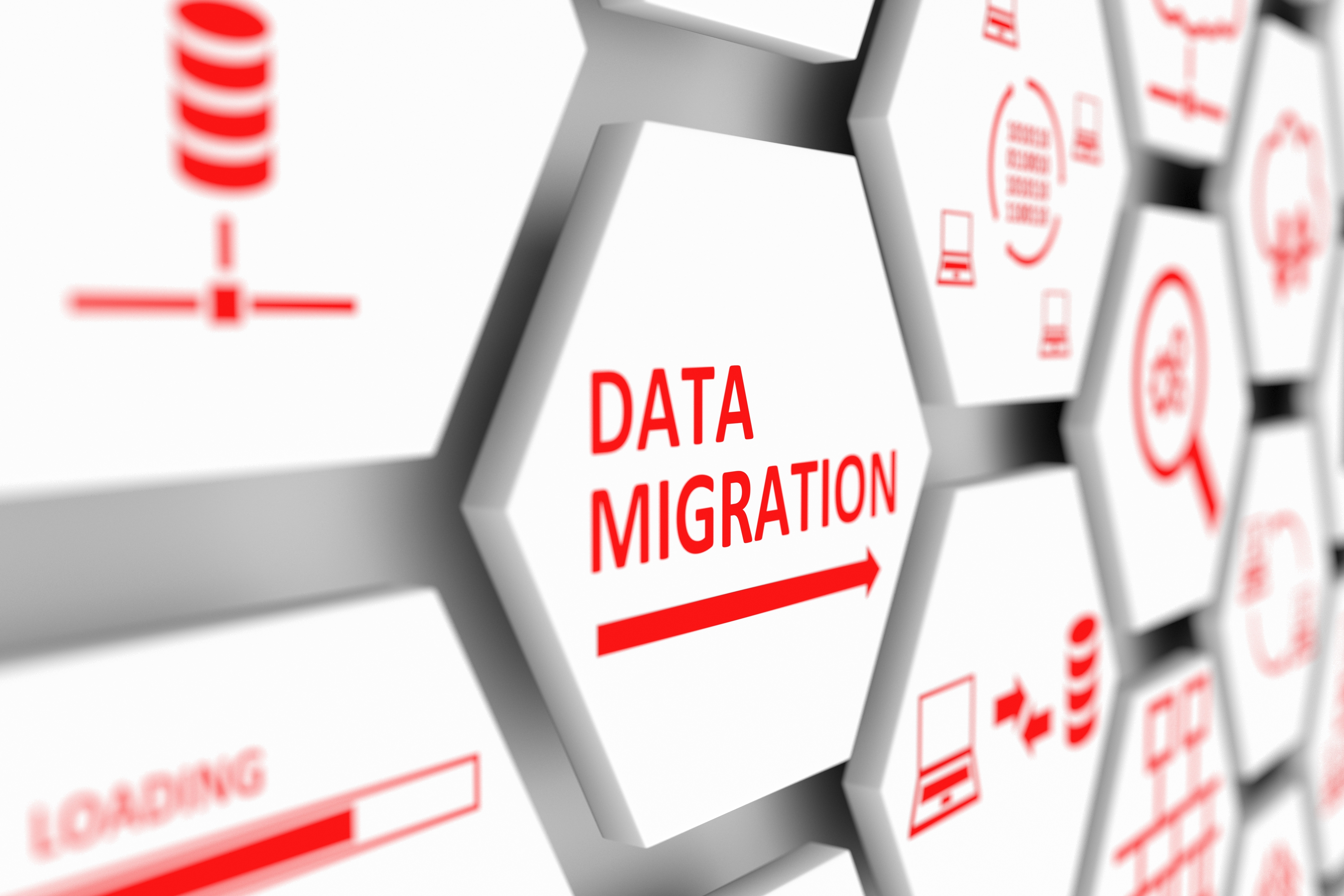
In the worst case, errors and inadequate data can lead to the AI software learning with incorrect assumptions and drawing useless conclusions. As AI is a black box, the models it uses to learn must be monitored and adapted. There is also the risk of overfitting: the AI transfers what it has learned during training to new data without evaluating it itself. In general, the introduction of AI requires a great deal of expertise and ties up IT capacities in the company. Data scientists are also not easy to recruit in today's specialist market. All of these reasons should not deter companies from getting involved in AI. However, it is important to consider and clarify these aspects in advance.
AI in manufacturing: Recommendations
AI-based software offers a range of opportunities to improve manufacturing processes, increase efficiency and retain customers through high product quality. These steps will help you prepare for the introduction:
Check how digital you already are and where you can further prepare manufacturing for the use of AI: What data do you already collect as standard? What does the hardware equipment with sensors, cameras and so on look like? Do you integrate the data collected on the shopfloor into the ERP and MES system? Are your employees connected to a connected worker platform via mobile devices?
Identify a suitable field of action: an AI project needs to be well prepared and ties up personnel and IT capacities. Check in which area the greatest effect can be expected or where you want to improve.
Prepare the introduction well: Scout the market and check whether you meet the data quality requirements of the respective provider. Take your time and only start a project once you have done your digital "homework". Also pay attention to the integration of the data into the business software. If necessary, hire specialist staff with AI skills and plan for sufficient capacity in your IT department.
Take your employees with you: Involve your colleagues on the shopfloor in the planning. Take the time to explain the benefits of the planned project and allay any fears and concerns. Make sure that all employees are familiar with the new processes, understand the purpose of the project and are willing to support the change.
Summary
Artificial intelligence is more than just automation. Thanks to self-learning algorithms, it is able to make predictions and derive recommendations for action. Manufacturing companies can take advantage of this in many areas - from order processing and predictive maintenance to quality control and energy management. As these are powerful solutions that are complex to implement, their introduction should be carefully considered. It is also important to clarify whether data can be recorded and consolidated in sufficient quantity and quality.
Markus Glotzbach
During his professional career, Markus gained deep insights at companies and large groups in different industries and knows the digitalization hurdles and problems on the shopfloor. Before joining Operations1, he studied International Management (MA) and worked in the Cloud Computing as well as SaaS telematics sector.